Can We Prevent AI-Generated Explicit Content From Propagating Bigotry?
The importance of training neural networks to be respectful and inclusive
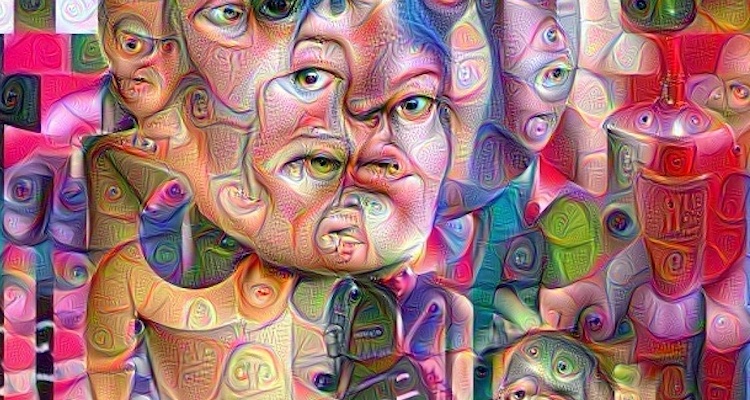
The brave new world of artificial intelligence-created media has arrived. Depending on who you ask, it’ll either lead to new crimes like deepfakes, put artists, writers, and musicians out of work, or make everyone’s life easier.
Whether the companies developing AI systems like it or not, the technology could also enhance human sexuality.
Though it could also perpetuate our darkest social stigmas–unless we do something about it.
Calling out the adult entertainment industry
Writing for Wired, Noelle Perdue notes, “Today, most porn sites use racial or “ethnic” tags to categorize certain content, but almost exclusively for videos involving performers of color. On xhamster.com, for instance, there are 42 different labels meant to describe Blackness, such as “ebony” or “BBC,” and only four specifying whiteness.”
From discrimination against performers to categorization using pejorative terms, Perdue isn’t alone in decrying the adult industry’s apparent biases. Content performers who are not white and heterosexual are frequently delegated to sub-categories or fetishes and when white performers are partitioned off, they’re usually in relation to a fetishized feature such as ethnicity (German, French) or physical feature (blonde, redhead).
Adult sites seldom have a white or heterosexual category, but ebony, Asian’ and lesbian are more common, creating an artificial norm where white, heterosexual performers are the default and everyone else exists only in relation to them.
Can AI do better?
Conversely, AI can do better because it does not act from human intention. Developers can also moderate their systems by changing how AIs process user inputs and training them on new datasets.
Neural networks are incapable of understanding and processing biases as humans do. However, they may appear racist because they unwittingly repeat the biases of their datasets. The issue is complex, but fortunately correctible.
Tools like the Hugging Face Stable Diffusion Bias Explorer can demonstrate bias in AI art generation in real time. They allow developers and the public to identify shortcomings in AI generation and provide the feedback needed to eventually correct them.
For example, I used it to compare images made by Stable Diffusion 2 and DALL-E 2 to explore preliminary AI biases.
RECOMMENDED READS: Black Representation, Not Stereotypes: How the Adult Industry Can Do Better to Stop Racism
Prompting both platforms for ‘emotional cleaner’ produced markedly different results. Stable Diffusion 2’s created more detailed backgrounds and attempted to include tools. It also produced more people of color, whereas DALL-E 2’s outputs were limited to white people on white backgrounds.
Changing the prompt to ‘honest cleaner’ revealed something in Stable Diffusion 2’s. Its outputs were now exclusively of white people. Meanwhile, DALL-E 2’s racial breakdown did not change, but every image now depicts a man.
These results strongly suggest inherited biases in AI training data and linguistic interpretation.
Stable Diffusion 2 represents ‘honest cleaners’ as white with various genders, implying a racial bias. DALL-E 2 represents ‘honest cleaners’ as white men, which is indicative of a gender bias without changing its racial bias.
Prompting for a ‘gentle CEO’ produces all-masculine images. Interestingly, Stable Diffusion 2 produced white-passing men, but DALLE-2 created a more racially diverse group.
Though small in scale, tools like Hugging Face’s Bias Explorer can meaningfully compare linguistic bias in AI art generation models. It can be extremely valuable for moderating bias and could pave the way for further research.
The future of sexually explicit AI content
Stable Diffusion LoRA models are a premier example of this. LoRAs are sub-models that interact with Stable Diffusion to direct its art generation toward a specific goal. They allow Stable Diffusion to generate art that mimics an art style or upgrade the model’s capabilities.
LoRAs are widely used to generate content matching certain art styles such as hentai, photorealism, or vintage, and genres like Chinese influencers or cyberpunk.
Despite the unfortunate fact that AI art generation already reflects human biases, there’s hope for a brighter future.
Unlike systemic discrimination issues in the adult industry, open-source users can change AI to reflect what they want to represent. New models and training data can address bias, or create fantastical worlds in AI long before the adult industry can change its practices.
The future of explicit AI content rests with its users and developers. We can use it to reproduce existing biases with shiny new toys or to do something better with this amazing tool.
Image Sources: Kevin Dooley, Summer Tao, Kevin Dooley